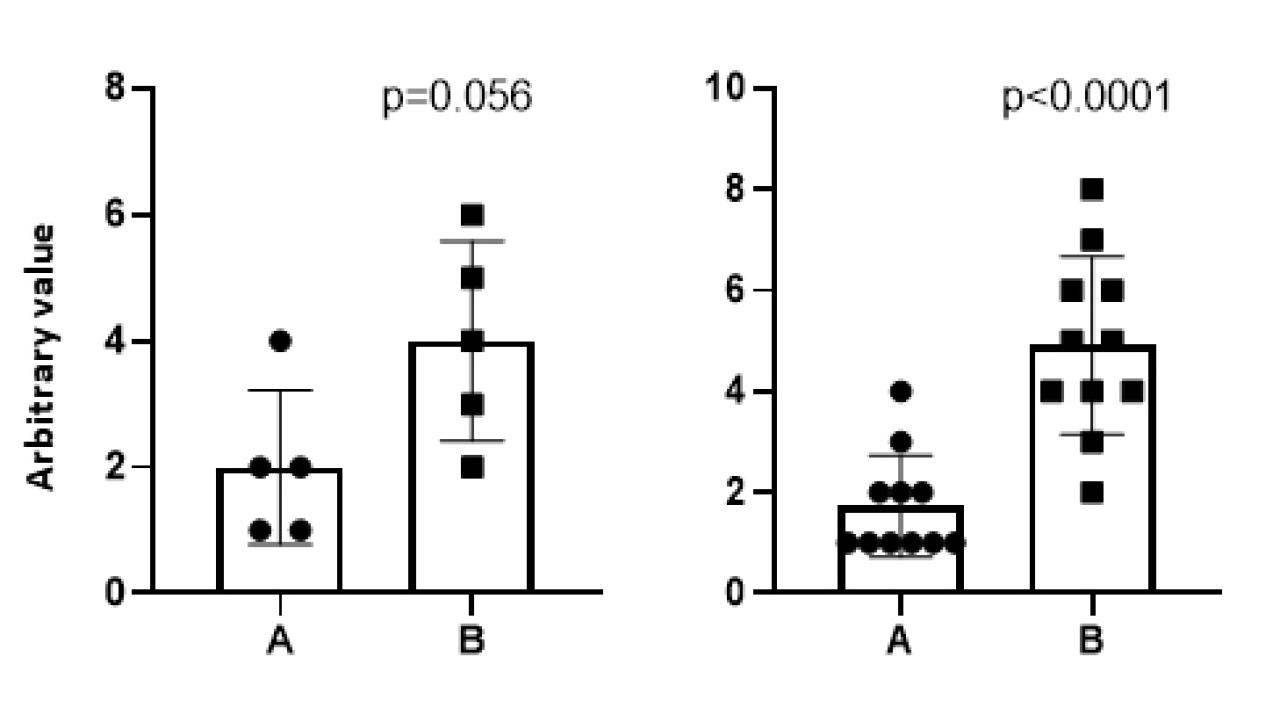
Why is Doing Good Science Difficult?
Why is Doing Good Science Difficult?
by Alan Nguyen
It has been a while since the last time I wrote something for the Endoplasmic Biotech-You-Learn blog. Since the last blog, I have been quite busy with doing experiments, analyzing data, and trying to tie up the last loose ends for my pending manuscripts. As you can probably imagine, I am nearing the end of my training as a Ph.D. student. Naturally, I am at the stage where I begin to look in retrospect at my journey through graduate school and reflect on the experiences that I have encountered. Some of my thoughts on those experiences include: “Why did I do that experiment that way?”; “I definitely could have done that better and saved myself so much time”; “I wish it turned out this way”; et cetera. Most of these thoughts revolve around the research work that I have conducted for my thesis, and one of the biggest things that I’ve learned during graduate school is this: doing good quality science is hard.
With the current COVID-19 pandemic, the world is focusing on scientists and researchers getting an effective treatment or vaccine against the SARS-CoV2 virus. You’ve probably seen people on social media rebuking the science, which can be due to misinformation. Sometimes, it could be due to contradicting claims amongst the scientific community, which can further deepen the public’s distrust toward scientists. As the saying goes, “the only thing that rebukes science is better science.” But how hard is it to do good science in the first place? Here, I thought it’d be a good idea to share my thoughts on why conducting thorough, high-quality science is difficult. By the time you finish reading this, I hope that you have a better understanding of why good science takes a long time to do.
Controls, Constants, and Variables
Every good experiment has the potential to yield data that is meaningful, even if the actual results mean that there are no differences between the groups being tested. How do you design a good experiment, though? One of the most fundamental things for a good experiment is to have proper controls. By definition, a control is “an observation designed to minimize the effects of variables other than the independent variable.” In other words, the control group or sample in the experiment ensures that the observed phenomena are indeed due to what is being tested.
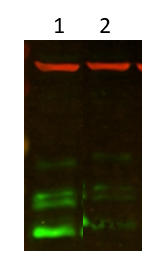
For example, let’s say you want to test whether or not a certain compound is effective at killing a specific bacterium. To set up this experiment, you have a number of petri dishes that have been inoculated with that bacterial strain. One group of petri dishes will be treated with the compound, and the other set of petri dishes will be part of the control group. In this case, the compound being tested is dissolved in a liquid (i.e. saline). For this experiment, a good control treatment would be the vehicle. In other words, the control group of petri dishes will be treated with the liquid used to dissolve the compound, minus the compound. This way, when you analyze the results, whatever differences you see on the petri dishes, it is most likely due to the compound, and not due to the liquid the compound is dissolved in.
Other controls in experiment are grouped as “technical controls”. An example of a technical control is the loading control in western blotting. Western blotting is used to detect proteins of interest and can be used to assess differences in the levels of protein expression. A scenario in which a loading control is used in western blotting is when a researcher is determining the levels of a phosphorylated protein between various groups. For this scenario, let’s pretend we’re looking at the levels of phospho-Erk. A loading control could be the quantification of total Erk. By determining the levels of total Erk, we can dismiss the notion that decreased levels of phosphorylated Erk is due to decreased levels of the protein itself. To get a better visual idea, you can refer to Figure 1.
In addition to proper controls, understanding experimental variables and how they can be sorted out later through data analysis is critical. The simplest experimental design, which I will describe here, only tests one variable at a time. Going back to the experiment with the efficacy of a compound being tested on bacterium, the variable is the treatment given to the bacteria. Hence, one must ensure that all the other conditions are the same for the petri dishes. These conditions can include incubation time, incubation temperature, volume of media used to inoculate the bacterium, the absolute number of bacteria used to inoculate the petri dishes, etc. By controlling all other conditions, one can make a surmise that the effects done on the bacteria was really from the tested compound, and not from a difference in the incubation temperature or something similar.
With the demands of designing a good experiment, it can be difficult to execute. There may be limitations in laboratory space, controlled environment facilities, equipment, or even in the costs of purchasing the necessary reagents. If one can overcome these limitations, or find suitable compromises, good science can be done. While these factors do play a major role in conducting good science, they are not everything.
Sample Size and Reproducibility
To design a good experiment, one must ensure that there is a large enough sample size to have confidence in the data. Simply put, the more samples you have per group, the better. Large sample sizes will address the variability within groups, especially if the study is centered on human subjects or specimens. As you already know, humans are much more diverse than laboratory animals, so it is essential to have a very large sample size to account for the diverse populations which innately introduces high variability.
Another reason to have a large sample size is to strengthen the statistical power of the study. Attaining statistical significance on a study means that the differences that are observed is not due to random chance. The larger the sample size, the easier it is to declare statistical significance when a difference is observed. A common method to determine statistical significance is by calculating the “p-value”, in which a p-value of less than 0.05 is considered significant. Figure 2 provides an example of how sample size can influence statistical significance. Also, ensuring that attention is paid to sample grouping (e.g. random block design) can be important for increasing statistical power.
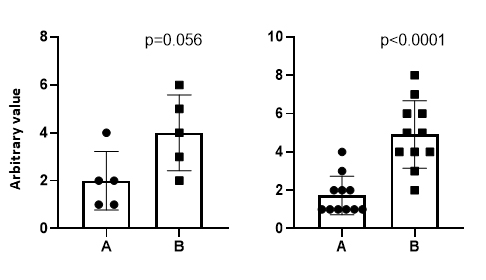
Okay, so now that we have a decent sample size, we only need to do the experiment, analyze the data and conclude the study, right? Well, not quite yet. Another characteristic of good science is that the study is reproducible. When one experiment is concluded, the next best thing to do is to repeat that experiment to confirm the results that were obtained from the first attempt. If the repeat experiment yields a different result, one must perform a few more repeats to confirm the true results. If the experimental results are the same the second time, it is common to repeat the experiment for a third time (if reagents and samples allow) to increase statistical power. Of course, there is also the possibility that the series of experiments will yield contradictory or inconclusive data, which will call into question the starting hypothesis.
Conclusion
To summarize, doing good science isn’t easy, but as long as you carefully plan your experiments, getting meaningful and strong data will be much more manageable. Some factors to having a good experiment is putting the proper control groups, recognizing all of the variables and having a good sample size. With a large sample size, you can easily attain statistical significance when a comparison is observed. Just remember that when you finish your first experiment, you’ll probably have to do it again to make sure the results of the first experiment are reproducible. Doing good science is an arduous process, so it’d be a good idea to have some of your lab mates help you during the experiments the lessen the technical burden (and it’d be more fun!). Good luck, and I wish you the best in conducting your experiments!